Unlock Text Analysis: Methods & Mixed Approaches
Is it truly possible to unlock the hidden narratives woven within the vast tapestry of text? Absolutely. The future of understanding lies in the potent fusion of technology and critical thought, where natural language processing dances with qualitative content analysis to reveal insights previously obscured.
The modern research landscape demands more than just surface-level readings. The ability to dissect, interpret, and synthesize textual data is becoming increasingly critical across disciplines. This article delves into the multifaceted dimensions of scholarly text analysis, showcasing how a synergistic blend of natural language processing (NLP) and qualitative content analysis (QCA) can unlock profound insights. Imagine being able to sift through mountains of documents, identifying subtle patterns, nuanced meanings, and hidden connections with unprecedented accuracy. This is the promise of combining these powerful methodologies.
The exploration begins by recognizing the inherent strengths and limitations of both NLP and QCA. NLP, with its algorithmic precision, excels at identifying keywords, sentiment, and relationships within text. It automates the initial stages of analysis, handling large datasets with ease and speed. However, NLP often struggles with context, irony, and the deeper, more subjective meanings that humans readily grasp. This is where QCA steps in, providing the interpretive framework necessary to understand the 'why' behind the 'what.' QCA, rooted in the humanities and social sciences, emphasizes the importance of human judgment, critical thinking, and a deep understanding of the subject matter. It allows researchers to delve into the nuances of language, uncovering hidden assumptions, biases, and power dynamics.
The true power of this approach emerges when NLP and QCA are integrated into a seamless workflow. NLP can be used to pre-process large volumes of text, identifying relevant passages and flagging potential areas of interest. This reduces the burden on human analysts, allowing them to focus their attention on the most critical aspects of the data. QCA then provides the interpretive lens through which these passages are examined, revealing the underlying meanings and implications. This iterative process, where NLP informs QCA and QCA refines NLP, leads to a richer, more nuanced understanding of the text.
Consider, for example, the analysis of political discourse. NLP can be used to identify the frequency with which certain keywords or phrases are used by different politicians, revealing their policy priorities and ideological leanings. However, QCA is needed to understand the context in which these words are used, the subtle rhetorical devices employed, and the overall message being conveyed to the public. By combining these methods, researchers can gain a more complete and accurate picture of the political landscape.
This integration extends beyond simply using NLP as a tool for QCA. It also involves adapting QCA methods to take advantage of the computational power of NLP. For example, researchers can use NLP to identify recurring themes or narratives within a text, and then use QCA to analyze the ways in which these themes are presented and framed. This allows for a more systematic and rigorous analysis of qualitative data, while also preserving the interpretive depth that is essential for understanding complex social phenomena.
- River Russell Deary Life Keri Russell Fame 2024 Update
- Selena Gomez Benny Blanco Wedding Bells Scoop Inside
The practical applications of this integrated approach are vast and varied. It can be used to analyze social media data, customer feedback, news articles, legal documents, and a wide range of other textual sources. It can help businesses understand their customers better, governments make more informed policy decisions, and researchers gain new insights into the human condition. The key is to recognize that NLP and QCA are not mutually exclusive alternatives, but rather complementary tools that can be used together to unlock the full potential of textual data.
In a world increasingly saturated with information, the ability to effectively analyze text is becoming an essential skill. By embracing the power of NLP and QCA, we can move beyond simply reading words to truly understanding the meanings and implications that lie within.
The synthesis of different research designs applying content analysis within mixed methods approaches is crucial for robust research. This chapter meticulously presents such a synthesis, illustrating how various research designs effectively incorporate content analysis within a broader mixed methods framework. This exploration acknowledges the limitations of relying solely on quantitative or qualitative methods, advocating for an integrated approach that leverages the strengths of both. The emphasis is on creating a holistic understanding of the research subject by combining different data collection and analysis techniques.
The chapter delves into specific examples of research designs, such as sequential explanatory designs, where quantitative data is initially collected and analyzed, followed by qualitative data collection and analysis to provide deeper insights and explanations. Conversely, sequential exploratory designs begin with qualitative data to explore a phenomenon, followed by quantitative data to test hypotheses or generalize findings. Concurrent designs, where quantitative and qualitative data are collected simultaneously, are also examined, highlighting the challenges and benefits of integrating data from different sources at the same time.
Each research design is presented with detailed case studies, demonstrating how content analysis is utilized within the specific methodological framework. For example, in a study examining public perception of climate change, quantitative data might be collected through surveys to measure awareness and attitudes. Content analysis could then be applied to media articles and social media posts to identify the dominant narratives and frames used to discuss climate change. The integration of these data sources provides a more comprehensive understanding of public perception, revealing both the extent of awareness and the underlying factors that shape attitudes.
The chapter also addresses the challenges of integrating content analysis within mixed methods research. These challenges include ensuring methodological rigor, managing data from different sources, and interpreting conflicting findings. Strategies for addressing these challenges are discussed, such as developing clear research questions, using triangulation to validate findings, and employing appropriate data analysis techniques.
Furthermore, the chapter emphasizes the importance of theoretical frameworks in guiding the integration of content analysis within mixed methods research. A strong theoretical framework provides a lens through which to interpret the data and helps to ensure that the research findings are meaningful and relevant. Examples of relevant theoretical frameworks include framing theory, agenda-setting theory, and social constructionism.
Ultimately, the chapter argues that the integration of content analysis within mixed methods research offers a powerful approach for addressing complex research questions. By combining the strengths of quantitative and qualitative methods, researchers can gain a more complete and nuanced understanding of the phenomena they are studying.
This review is grounded in a meticulously selected strategic sample of cases and studies. It serves to articulate a cohesive view of research areas that, on the surface, may appear disparate and unconnected. The selection process prioritizes studies that demonstrate innovative applications of mixed methods research, combining quantitative and qualitative approaches to address complex research questions. The review aims to bridge gaps between seemingly unrelated fields, highlighting the common methodological challenges and opportunities that arise when integrating different research traditions.
The strategic sampling approach involves identifying key studies that represent a range of disciplines, methodological approaches, and research topics. Criteria for inclusion include the rigor of the research design, the clarity of the research findings, and the relevance of the study to the broader themes of mixed methods research. The selected cases and studies are then analyzed in detail, focusing on the specific ways in which quantitative and qualitative methods are integrated, the challenges encountered, and the lessons learned.
One of the key goals of this review is to demonstrate the versatility of mixed methods research. The selected cases and studies showcase how this approach can be applied to a wide range of research questions, from understanding the impact of social media on political polarization to evaluating the effectiveness of educational interventions. By highlighting the diverse applications of mixed methods research, the review aims to encourage researchers to consider this approach as a viable option for addressing their own research questions.
The review also examines the specific techniques used to integrate quantitative and qualitative data in the selected cases and studies. These techniques include triangulation, where data from different sources are compared to validate findings; sequential designs, where quantitative and qualitative data are collected in separate phases; and concurrent designs, where quantitative and qualitative data are collected simultaneously. The strengths and limitations of each technique are discussed, providing researchers with guidance on how to choose the most appropriate approach for their own research.
Furthermore, the review addresses the ethical considerations that arise when conducting mixed methods research. These considerations include ensuring informed consent, protecting the privacy of participants, and managing potential conflicts of interest. The review emphasizes the importance of adhering to ethical principles throughout the research process, from the design of the study to the dissemination of the findings.
In conclusion, this review offers a valuable resource for researchers interested in learning more about mixed methods research. By providing an articulated view of disparate and unconnected research areas, the review demonstrates the versatility and potential of this approach. It also offers practical guidance on how to design and conduct rigorous mixed methods studies, while addressing the ethical considerations that arise in this type of research.
This chapter provides a comprehensive overview of how mixed method approaches can optimize the use of content analysis, specifically to analyze the impact of communication on public opinion and to rigorously validate research findings. It underscores the synergistic relationship between quantitative and qualitative data, illustrating how their integration yields a deeper, more nuanced understanding of complex phenomena. The chapter emphasizes the strategic application of content analysis within mixed methods designs to enhance the validity and reliability of research outcomes.
The analysis of communication effects on public opinion is a central focus. Content analysis, when combined with quantitative methods such as surveys and statistical modeling, allows researchers to examine the relationship between media messages and public attitudes. For example, content analysis can be used to identify the dominant themes and frames used in news coverage of a particular issue, while surveys can measure public awareness and attitudes towards that issue. By integrating these data sources, researchers can assess the extent to which media messages influence public opinion.
The chapter also explores the use of mixed methods approaches to validate research findings. Triangulation, a key principle of mixed methods research, involves using multiple data sources and methods to confirm or disconfirm findings. Content analysis can play a crucial role in triangulation, providing qualitative data that can be compared with quantitative data to assess the validity of research outcomes. For example, if a survey finds that a particular intervention is effective in improving student achievement, content analysis can be used to examine classroom observations and student work to identify the mechanisms through which the intervention is working.
The chapter also addresses the challenges of using mixed methods approaches in content analysis research. These challenges include ensuring methodological rigor, managing data from different sources, and interpreting conflicting findings. Strategies for addressing these challenges are discussed, such as developing clear research questions, using appropriate sampling techniques, and employing robust data analysis methods.
Furthermore, the chapter highlights the importance of theoretical frameworks in guiding the use of mixed methods approaches in content analysis research. A strong theoretical framework provides a lens through which to interpret the data and helps to ensure that the research findings are meaningful and relevant. Examples of relevant theoretical frameworks include agenda-setting theory, framing theory, and social constructionism.
In conclusion, this chapter offers a valuable resource for researchers interested in learning more about how mixed method approaches can optimize the use of content analysis. By providing a comprehensive overview of the key principles, techniques, and challenges involved, the chapter equips researchers with the knowledge and skills they need to conduct rigorous and impactful mixed methods research.
The two distinct traditions of content analysis qualitative and quantitative possess a rich history within the social sciences and related disciplines. However, despite their individual strengths and long-standing presence, the potential for synergistic integration within a mixed methods framework remains largely unexplored. This section delves into the historical development of both qualitative and quantitative content analysis, highlighting their respective contributions and limitations, while advocating for a more unified approach that leverages their complementary strengths.
Quantitative content analysis, with its roots in positivism, emphasizes objectivity, reliability, and generalizability. It involves systematically counting and categorizing textual data to identify patterns and trends. This approach is particularly well-suited for analyzing large datasets and generating statistical findings. For example, researchers might use quantitative content analysis to track the frequency with which certain keywords appear in news articles over time, or to compare the representation of different groups in media coverage.
Qualitative content analysis, on the other hand, adopts a more interpretive and holistic approach. It focuses on understanding the meanings, contexts, and nuances of textual data. This approach is particularly useful for exploring complex social phenomena and generating rich, descriptive insights. For example, researchers might use qualitative content analysis to examine the ways in which individuals make sense of their experiences, or to analyze the rhetorical strategies used in political discourse.
Despite their different philosophical underpinnings and methodological approaches, qualitative and quantitative content analysis share a common goal: to systematically analyze textual data to gain insights into the social world. However, each approach has its own limitations. Quantitative content analysis can be criticized for being overly reductionistic and for neglecting the context in which textual data is produced and consumed. Qualitative content analysis, on the other hand, can be criticized for being subjective and lacking generalizability.
The integration of qualitative and quantitative content analysis within a mixed methods framework offers a promising way to overcome these limitations. By combining the strengths of both approaches, researchers can gain a more comprehensive and nuanced understanding of complex social phenomena. For example, researchers might use quantitative content analysis to identify patterns and trends in a large dataset, and then use qualitative content analysis to explore the meanings and contexts of those patterns and trends.
The potential benefits of this integrated approach are significant. It allows researchers to generate more robust and valid findings, to explore complex research questions in greater depth, and to bridge the gap between quantitative and qualitative research traditions. However, the integration of qualitative and quantitative content analysis also presents a number of challenges. These challenges include ensuring methodological rigor, managing data from different sources, and interpreting conflicting findings. By addressing these challenges, researchers can unlock the full potential of mixed methods content analysis and contribute to a more comprehensive and nuanced understanding of the social world.
The term "method" refers to a more technical level, encompassing the specific ways in which data are collected, processed, analyzed, and presented in a research study. It represents the practical implementation of a research design, detailing the concrete steps taken to gather and interpret information. Understanding the various methods available is crucial for conducting rigorous and impactful research, as the choice of method directly influences the quality and validity of the findings.
At its core, a method is a systematic procedure for gathering and analyzing data. It provides a framework for researchers to follow, ensuring that the research process is transparent, replicable, and reliable. The selection of appropriate methods depends on the research question, the nature of the data being collected, and the goals of the study. Researchers must carefully consider the strengths and limitations of each method before making a decision.
The process of data collection is often the first step in the research process. It involves gathering information from various sources, such as individuals, documents, or observations. Common data collection methods include surveys, interviews, focus groups, experiments, and document analysis. Each method has its own advantages and disadvantages, and the choice of method depends on the specific research question and the type of data being sought.
Once data has been collected, it must be processed and analyzed. Data processing involves cleaning, organizing, and transforming the data into a usable format. Data analysis involves using statistical or qualitative techniques to identify patterns, trends, and relationships in the data. The choice of data analysis method depends on the type of data being analyzed and the research question being addressed.
Finally, the results of the data analysis must be presented in a clear and concise manner. Data presentation involves using tables, graphs, and other visual aids to communicate the research findings to a wider audience. The goal of data presentation is to make the research findings accessible and understandable to readers, allowing them to draw their own conclusions about the implications of the research.
In conclusion, the term "method" encompasses the technical aspects of research, including data collection, processing, analysis, and presentation. The selection of appropriate methods is crucial for conducting rigorous and impactful research, and researchers must carefully consider the strengths and limitations of each method before making a decision.
Examples of methods include, but are not limited to, tests, interviews, and questionnaires. These tools are fundamental in gathering data across various research disciplines, each offering distinct advantages and tailored to specific research objectives. Understanding the nuances of each method is crucial for researchers seeking to collect relevant, reliable, and valid information.
Tests, for instance, are standardized procedures designed to measure specific skills, knowledge, or abilities. They can range from simple multiple-choice quizzes to complex performance-based assessments. Tests are often used in educational settings to evaluate student learning, in employment settings to assess job candidates, and in psychological research to measure personality traits or cognitive abilities. The key to a good test is its validity and reliability; it must accurately measure what it is intended to measure, and it must produce consistent results over time.
Interviews, on the other hand, involve direct interaction between a researcher and a participant. They can be structured, semi-structured, or unstructured, depending on the level of standardization. Structured interviews follow a pre-determined set of questions, while semi-structured interviews allow for some flexibility and follow-up questions. Unstructured interviews are more conversational and exploratory, allowing the participant to guide the discussion. Interviews are particularly useful for gathering in-depth information about people's experiences, perspectives, and beliefs.
Questionnaires are another common data collection method. They typically consist of a series of written questions that participants answer on their own. Questionnaires can be administered online, in person, or through the mail. They are often used to collect data from large samples of people, as they are relatively inexpensive and easy to administer. Questionnaires can include a variety of question types, such as multiple-choice questions, rating scales, and open-ended questions.
Each of these methods has its own strengths and limitations. Tests are useful for measuring specific skills or knowledge, but they may not capture the full complexity of human behavior. Interviews provide rich, in-depth data, but they can be time-consuming and expensive to conduct. Questionnaires are efficient for collecting data from large samples, but they may not allow for follow-up questions or clarification.
Researchers must carefully consider the strengths and limitations of each method when designing a research study. The choice of method should be guided by the research question, the type of data being sought, and the resources available.
- Stream It All Movies Web Series Sports More Online Now
- Unveiled Oak Islands Secrets Truth Finally Found New Research
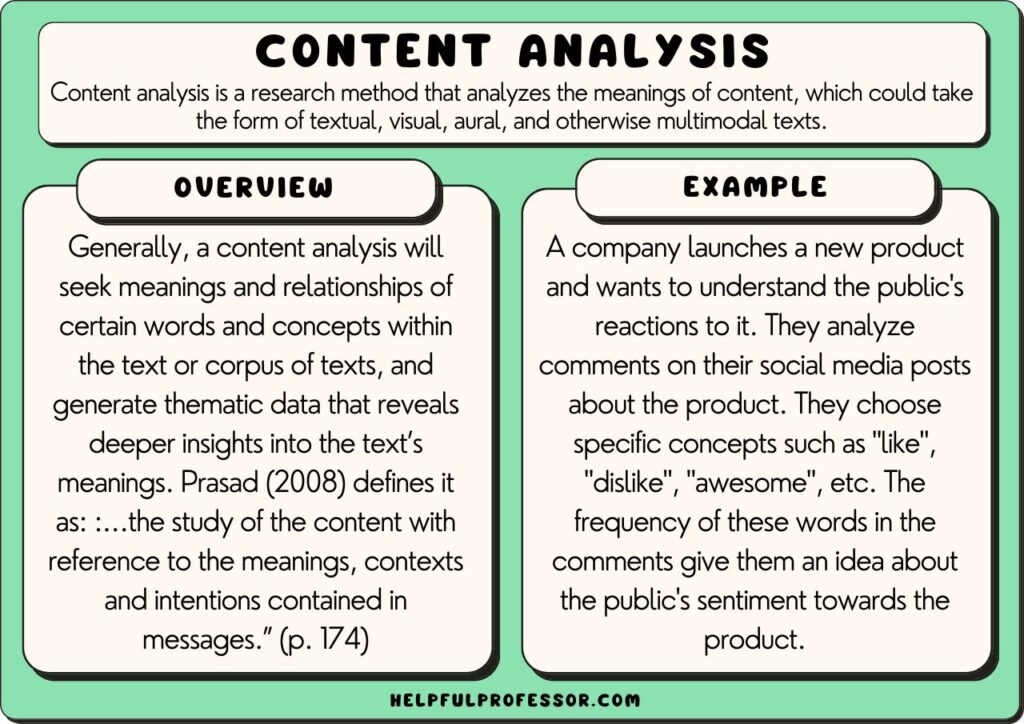
10 Content Analysis Examples (2024)
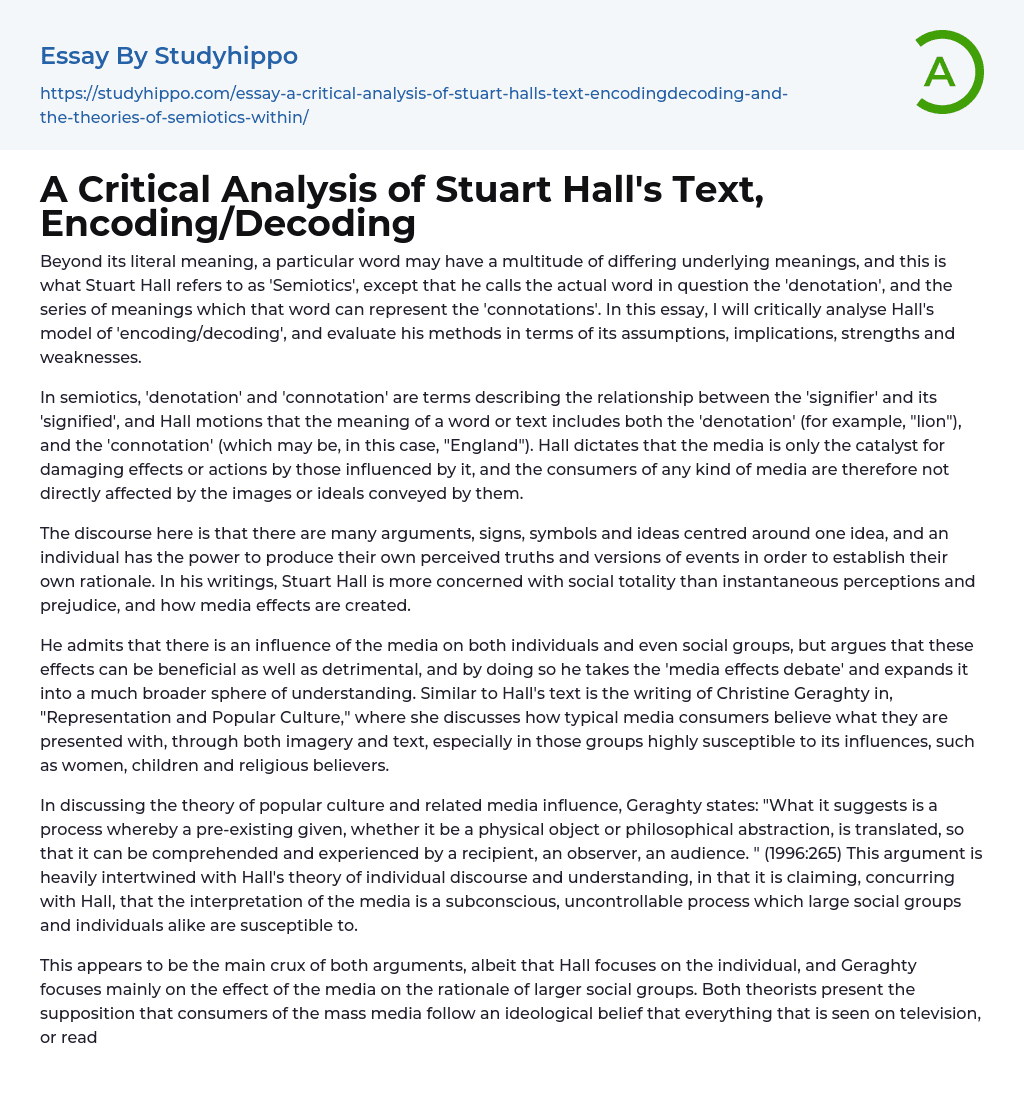
A Critical Analysis of Stuart Hall’s Text, Encoding/Decoding Essay
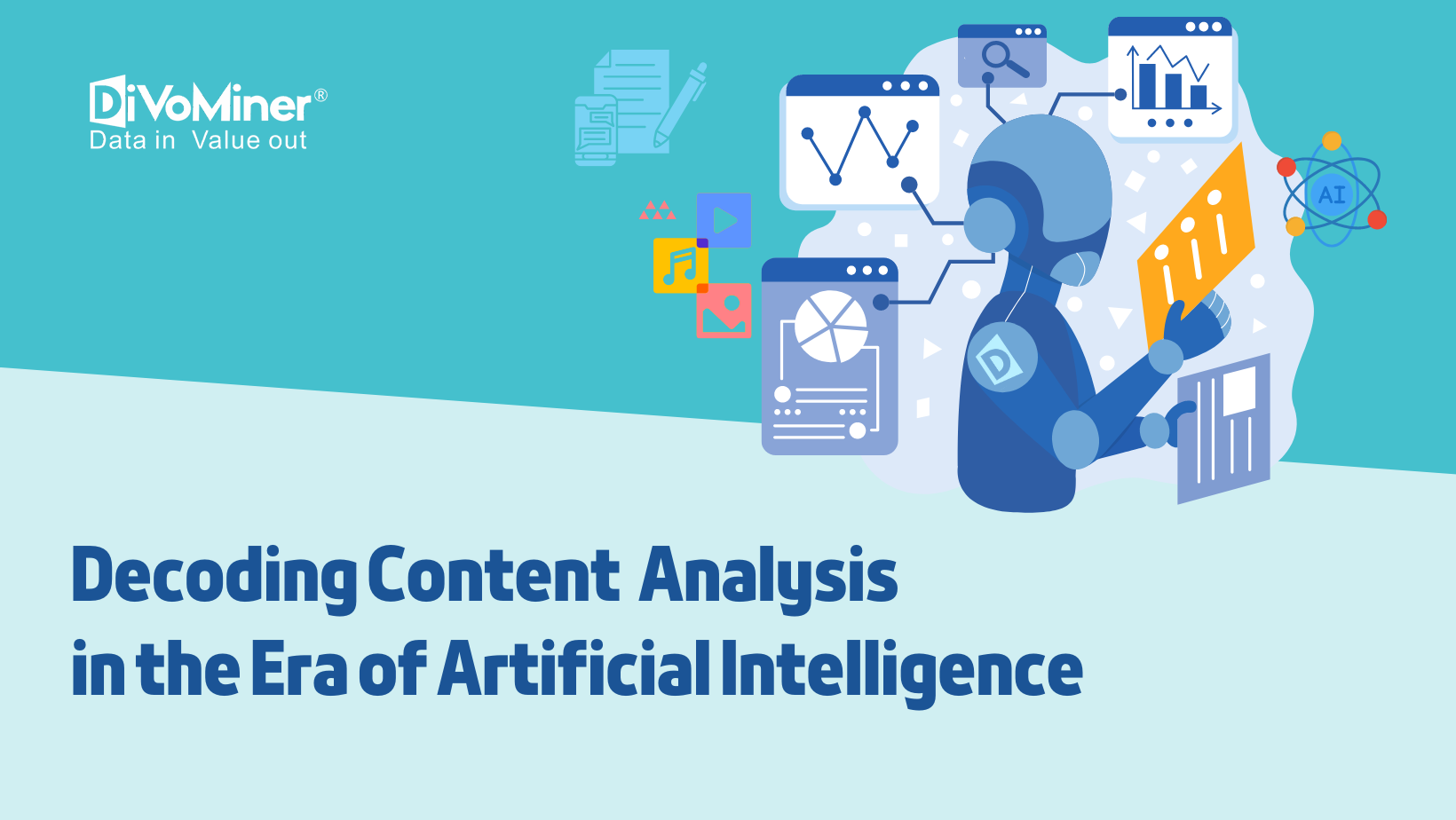
Decoding Content Analysis In The Era Of Artificial Intelligence